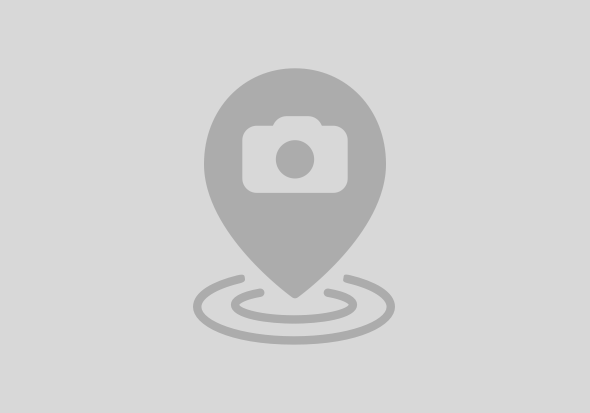
This guide will explain how one can enable observability on NSO and process the data with a data science mechanism with the help of Splunk. We will separate the guide into two parts on two different blog post
Part 1 will focus on how to enable observability and start data collection from NSO. More specifically, set up the Observability Exporter to stream the time consumption data per transaction to Splunk. At the same time, stream memory consumption data for "ncs.smp" or even Java and Python with CollectD. Eventually, how one can perform feature-based performance measurements on memory and time consumption and stream the data to Splunk for further processing.
Part 2 will focus more on data processing, visualization, and machine learning prediction. At the same time, it will discuss how to use prediction data and take action with it. For more detail on part 2, we will discuss in the next blog post.
For part 1, we will introduce how one can set up the environment below to start the data collection from NSO and feature-based performance measurement testbed (Performance Data Collector). In the diagram below, one can see the green box is the Data Collection Section. Inside the green box, there are two different data sources for the OTEL Collector (grey box). The Operating System (OS) and NSO (Blue Box) will stream the live data on memory consumption over time and time consumption per transaction to the Collector. At the same time, the Performance Data Collector will send the experimental performance measurement per feature to the collector. By performance measurement, what we mean is the time and memory consumption over an independent variable X of a specific feature. For example, in this guide, we will use the time and memory consumption of the order by user list when the list length keeps growing. In this case, my X is the list length and the data I stream to the Collector is the time and memory consumption per list length(X). In fact, the Collector for Performance Data Collector and NSO/OS are different Collector. For simplification purposes, we only plot one Collector in the diagram below.
When the Collector receives the data on the receiver side, it exports the data into standardized OTEL format and streams it to Splunk (Orange Box). Splunk stores the data and allow Splunk users to proceed with data processing with it. We will further expand this diagram with Data Processing and Visualization in Part 2.
This article comes with three code examples. We strongly recommend you clone these examples and go through the Readme before proceeding further. In this case, you can follow our journey through this guide by also trying to do it yourself. Learning by doing is always easier than just reading.
The Splunk NSO Integration Example correlates to the blue boxes in the diagram in the Introduction while Lux-based Performance Data Collector is the grey box for Performance Data Collector.
In our setup, we use Splunk Enterprise in Container - https://hub.docker.com/r/splunk/splunk/. However, the example above does not include Splunk Enterprise. One needs to set up separately and obtain the IP address of your Splunk Instance. If one needs more features or longer development time, applying for a Splunk Enterprise Developer License is also a good choice - https://dev.splunk.com/enterprise/dev_license.
It is also a good idea to take a look at the NSO Observability Exporter guide - https://developer.cisco.com/docs/nso/observability-exporter/ and OTLP/HTTP Exporter Readme - https://github.com/open-telemetry/opentelemetry-collector/blob/main/exporter/otlphttpexporter/README.md before proceed further. This will give you a basic understanding of what we will do next.
In this chapter, we will start with configuring Collector and Splunk to receive data on memory and transactional time consumption with CollectD, Observability Exporter (OE), and Performance Data Collector. The observability Exporter (OE) and Performance Data Collector will stream data to the opentelemetry-collector - https://github.com/open-telemetry/opentelemetry-collector-contrib before the collector exports the data to Splunk. However, CollectD will directly send data to Splunk, and Splunk will parse and process the data with “Splunk Add-on for Linux”.
The first step is to set up the Collector receiver and exporter. Inside the repository above, one can find the example setup at “run/otel-collector-config.yml”.
On the receiver side one needs to define what protocol will the Collector receive from on what IP address and port number. In the example below, we set up grpc to receive on <Collector IP> at port 4317 while http is on the same IP address but on port 4318. This means we need to set up the same destination IP address and port on the NSO and CollectD side later.
receivers:
otlp:
protocols:
grpc:
endpoint: "<Collector IP>:4317"
http:
endpoint: "<Collector IP>:4318"
After data arrives at the collector, we also need to tell the collector where to export the data towards in the collector. We can define two kinds of exporters – events(traces) and metrics. These two exporters export data to different URLs. Therefore, we need to configure them separately. More specifically, “events” will export data to the Splunk via "/v1/traces" while the “metrics” export via “/v1/metrics”. In the Observability Exporter example, we will export both events and metrics. However, we will only export the events in the CollectD example. In the example below, we list out the mandatory configuration needed for the export to work. There is also another optional configuration listed in the OTLP/HTTP Exporter Readme which we will not discuss here. For this specific exporter configuration example is expecting the Splunk to receive via HTTP Event Collector (HEC) without TLS. The setup guide of HEC can be found in the link below
https://docs.splunk.com/Documentation/SplunkCloud/latest/Data/UsetheHTTPEventCollector
exporters:
splunk_hec/events:
# Splunk HTTP Event Collector token.
token: "<HEC Token>"
# URL to a Splunk instance to send data to.
endpoint: "http://<Splunk IP>:8088/services/collector"
# Optional Splunk source: https://docs.splunk.com/Splexicon:Source
source: "otel-events"
# Optional Splunk source type: https://docs.splunk.com/Splexicon:Sourcetype
sourcetype: "otel-events"
# Splunk index, optional name of the Splunk index targeted.
index: "events"
# Whether to disable gzip compression over HTTP. Defaults to false.
disable_compression: false
# HTTP timeout when sending data. Defaults to 10s.
timeout: 10s
# Whether to skip checking the certificate of the HEC endpoint when sending data over HTTPS. Defaults to false.
# For this demo, we use a self-signed certificate on the Splunk docker instance, so this flag is set to true.
tls:
insecure_skip_verify: true
The token parameter is obtained from the Splunk HEC under the “Token Value” after the configuration tutorial is complete. For example, the diagram below shows where I obtained the token from the example above.
The endpoint is the destination URL of your Splunk Instance. It is usually under the following format http://<Splunk IP>:8088/services/collector and the default port for HEC is 8088. If you want to use another port, you can modify it in the global setting under HTTP Port Number like the screenshot below.
The source type defines how Splunk will parse your data. Since we are using the OTEL Events format, we will use “otel-events”. For OTEL metrics, one can use “otel-metrics”. During the setup wizard of “New Token”, you probably not going to find the “otel-events”. You can choose “Automatic” during the setup and edit the configuration after the setup wizard. In the “Edit”, one can find the source type can be entered via the text box rather than choosing from the existing list like the diagram below.
In this case, one can enter the “otel-events” as source type. For the “source”, one can override the existing value during the setup wizard at the first step inside “Source name override” like the screenshot shown below.
The index is used to categorize the data that came in. For example, to separate the data from Performance Data Collector and Observability Exporter we set different indexes. In this case, we can choose which data to choose from during the Data Processing. You can create a custom index via Settings -> DATA -> Indexes -> New Index.
Then Click on “New Index” on the top right corner of the page. The two most important field is the “Index Name” and “Index Data Type”. “Index Name” must be the same name as you configured under the “index” field in the collector. Afterward, one needs to choose the correct “Index Data Type” because Splunk will enable different filter queries during the Data Processing and Visualization stage afterward.
Afterward, back to the HEC guide and select the created index in the “Select Allowed Indexes” field as shown below. The selected index will show up in the “Selected Item” box. Make sure also choose the Default Index as the primary index you want the current HEC to use.
The final configuration overview on the Splunk side can be found below. This screen is open by clicking on the “Edit” button below. Make sure to compare your one with your configuration in the collector and make sure it is consistent. In my configuration overview, I also added the “events_perf” index to ask the same HEC collector to receive both data from the Observability Exporter(events) and Performance Data Collector(events_perf).
Eventually, we also set the “insecure_skip_verify” under TLS to true even if we use only HTTP. Just make sure TLS Host Verification will not block our way with some errors. At the same time, configure a timeout to prevent the data export session from hanging forever.
Splunk has multiple guides explaining how to set up CollectD with Splunk on the Splunk side. In this chapter, we will summarize the steps and direct the reader toward the correct Splunk Guide.
The result of doing the step above is to gain access to the “linux:collectd:http:json” source type. Afterwards, one can configure the following HEC settings. The “ncssmp” index is the dedicated index created for CollectD data.
At this stage, we have the Receiver side ready to receive data from the Data Streamer. In this chapter, one can find how to set up data streaming via Collector and Splunk Directly through Add-on. For Observability Exporter and CollectD, one can find the setup method with both Native Install on the barebone machine or via Containerized NSO.
Both the Observability Exporter and Performance Data Collector stream to the Collector before the Collector exports data to Splunk. In this chapter, we will focus on how to set up NSO and Performance Data Collector to stream data to the Collector.
Observability Exporter (OE) stream Transactional Time Consumption by sending the progress trace in OTEL format to the Collector. At the same time, some other metrics that Splunk can be used for some metrics measurement. To set up the connection between the NSO OE to the Collector, the following configuration under “progress export” is needed. One thing to take in mind is that “progress export” is a hidden configuration. So, make sure you “unhide debug” before the configuration.
admin@ncs# unhide debug
admin@ncs# show running-config progress
progress export enabled
progress export otlp host <Collector IP>
progress export otlp port 4318
progress export otlp transport http
progress export otlp metrics host <Collector IP>
progress export otlp metrics port 4318
The configuration is under “otlp” since we are sending data to the OTLP(OpenTelemetry Protocol) to the OTEL(open-source observability framework) Collector that speaks OTLP. Under “otlp” we set up the host IP address as the remote Collector address with the same port we configured as HTTP Receiver on the Collector side. Then we set the transport as HTTP. At the same time, set metrics exporting by configuring the host IP and port towards the same collector. The available metric from NSO side can be found in the yang model - "$NCS_DIR/src/ncs/yang/tailf-ncs-metric.yang" while the trace OE send is from progress trace.
Performance Data Collector is aimed at collecting Performance-related data (memory and time consumption) under a specific feature per Independent Value X. The farmwork is based on Erlang Testing Framework Lux - https://github.com/hawk/lux. The Lux framework is network automation friendly due to the design of pattern matching. It sends a command (with ! header) and sees if the reply output is the same as defined(with ? or ??? header). For example,
[shell trigger]
!commit
???Commit complete.
It also allows to start of multiple shell windows to perform multiple tasks concurrently. The example above is called the “commit” command and expects the output from NSO as “Commit complete.” Inside the trigger shell.
The diagram below shows a higher-level overview of how the Lux is used in Performance Data Collector. The Interation Script trigger Lux per X with ITERATION apart until reaches the MaxX. ITERATION and MaxX are variables that are defined in the Makefile.
Inside each Lux script iteration, it begins with the startup of the NSO in the “shell nso”.
[shell nso]
[timeout infinity]
[progress "\nStarting up NSO. This will takes a while....\n"]
!make clean_cdb clean_logs start_nso && echo ==$$?==
?==0==
[timeout 30]
[progress "\nPreparing....\n"]
!make cli-c
?$oper_prompt
!config
?$cfg_prompt
!services global-settings service-callback-timeout 6000
?$cfg_prompt
!commit
???Commit complete.
Then enter the ncs_cli in “shell trigger” and start preparation before the data measurement.
[shell trigger]
[progress "\Prepare\n"]
# Preparations
!make cli-c
?$oper_prompt
!config
?$cfg_prompt
#Create Service
!predictive_service test max-length $X
?$cfg_prompt
Afterward, start the data measurement by calling the “data_collect.sh” in the “shell collect”.
[shell collect]
[progress "\nStart Collecting Data for X=$X....\n"]
!make collect && echo ==$$?==
?==0==
Eventually, trigger the command that one wants to measure the performance
[shell trigger]
[timeout infinity]
[progress "\Triggering....\n"]
# The operation you want to collect data on
!commit
???Commit complete.
and stop the data collection with few initial data processing (for example take average).
[shell collect]
[progress "\nStop Collecting and Start Processing Data....\n"]
!make X=$X stop_collect && echo ==$$?==
?==0==
For the sequence above in the Lux test cases, we call it from X=0 to X=MaxX with INTERVAL apart in the "trigger.sh". “MaxX“ and “INTERVAL” are defined in the Makefile at the root path of the “Performance Data Collector”. At the same time, the data is streaming to the Collector per round via “send_splunk.sh” which is called the opentelemetry API in “lib/splunk.py”. “lib/splunk.py” sends the data to the Collector via trace. Inside the “send_trace” function one can modify the data name by modifying the parameter name behind “current_span.set_attribute”. At the moment, we set send memory data as “mem” and time data as “time” per Independent Variable X as “x”.
def send_trace(tracer,msg,mem,time,kvs=""):
with tracer.start_as_current_span("span-name") as current_span:
print("sending: "+msg + " "+kvs+" " + mem+" " + time)
#current_span = trace.get_current_span()
#current_span.add_event(msg)
current_span.set_attribute("x", msg)
current_span.set_attribute("mem", mem)
current_span.set_attribute("time", time)
In the packages folder of the “Performance Data Collector”, we provide a sample service that takes in the length of the order-by-user list and creates this specific length of the order-by-user list via FastMap via the command below.
predictive_service test max-length <length>
In this case, we can use this testbed to measure the time and memory consumption of various lengths of the order-by-user list from 0 to “MaxX” with “INTERVAL” apart.
From the CollectD side, one needs to start with installing the CollectD agent via the following command.
sudo apt-get install collectd
sudo service --status-all | egrep "collectd|apache2"
systemctl restart collectd.service
systemctl status collectd.service
Afterward, we need to configure the CollectD configuration file in “/etc/collectd/collectd.conf”. First of all, we need to let CollectD monitor the “ncs.smp” process. One can also use” java” for "java-vm" or “python” for "python-vm" here. What we are actually doing is enable the processes plug-in and configure the process to monitor under the “Process” configuration.
LoadPlugin processes
<Plugin processes>
Process "ncs.smp"
</Plugin>
Then configure the data export by enabling the “write_http” plug-in. Inside the “write_http” configuration, we set the target URL as the IP address of Splunk via port 8088. However, since the data does not go through the collector now, we set the data as raw data by specifying the “raw” path behind the /collector/. Eventually, define the HEC Token under the parameter “channel” in the URL and under the Authorization Header behind the Splunk keyword. Eventually, we set the exporting format as JSON and allowed metrics export like what we did with NSO Observability Exporter before.
LoadPlugin write_http
<Plugin write_http>
<Node "splunk">
URL "http://<Splunk IP>:8088/services/collector/raw?channel= <HEC Token>"
Header "Authorization:Splunk <HEC Token>"
Format "JSON"
Metrics true
StoreRates true
</Node>
</Plugin>
Afterward, restart the CollectD to load the config we changed above.
systemctl restart collectd.service
systemctl status collectd.service
An example CollectD configuration file can be found in the “collectd/collectd.conf” in the code example provided above. One can modify the example and copy it to the “/etc/collectd/collectd.conf”.
The log of the Collector can be collected via the command below.
$ docker logs <container id> -f
If the data is correctly accepted by the Collector, the docker logs of the Collector should remain silent. Otherwise, it will either throw an exception or have an Error log showing what has gone wrong.
At the same time, if Splunk successfully receives the data, the event count under a specific index under Settings -> Indexes will grow from 0 to a larger number like the one shown below.
These two methods can help you define if your issue is between NSO and the Collector or between Collector and Splunk.
You must be a registered user to add a comment. If you've already registered, sign in. Otherwise, register and sign in.
Find answers to your questions by entering keywords or phrases in the Search bar above. New here? Use these resources to familiarize yourself with the NSO Developer community: