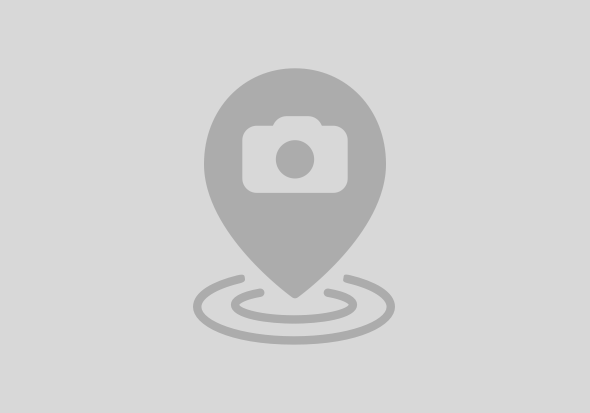
- Subscribe to RSS Feed
- Mark as New
- Mark as Read
- Bookmark
- Subscribe
- Printer Friendly Page
- Report Inappropriate Content
The Model Context Protocol (MCP) is an open standard that provides a universal way to connect AI models and agentic applications to various data sources and tools. It's how AI applications and applications can supply context (documents, database records, API data, web search results, etc.) to AI applications. This capability is very powerful, but it also raises important cybersecurity-related questions.
Note: I wrote a detailed article titled “Integrating Agentic RAG with MCP Servers: Technical Implementation Guide”
When AI assistants gain access to sensitive files, databases, or services via MCP, organizations must ensure those interactions are secure, authenticated, and auditable.
The MCP architecture (host, client, servers) inherently creates defined points where security controls can be applied. Let’s explore how MCP can be leveraged for security purposes (from securing model interactions and logging their actions, to guarding against adversarial inputs and ensuring compliance with data protection).
Overview of MCP and Its Security Architecture
MCP follows a client-server model with clear separation of roles.
An MCP Host (the AI application or agent) connects via an MCP Client library to one or more MCP Servers.
Each server exposes a specific set of capabilities (such as reading files, querying a database, or calling an API) through a standardized protocol. This is why people refer to MCP as the “USB-c port for AI applications”.
By design, this architecture introduces security boundaries: the host and servers communicate only via the MCP protocol, which means security policies can be enforced at the protocol layer. For example, an MCP server can restrict which files or database entries it will return, regardless of what the AI model requests. Likewise, the host can decide which servers to trust and connect to. This clear delineation of components makes it easier to apply the Zero Trust principle (treating each component and request as potentially untrusted until verified).
Security Considerations when Using MCP
The following table outlines a few security when implementing MCP.
Security Concern |
Description |
Unmonitored Access |
Without proper visibility, AI assistants could access or modify sensitive data without detection. A compromised prompt or malicious instruction might extract confidential information using legitimate MCP connections. |
Lack of Built-in Approval Workflows |
Standard MCP implementations do not include built-in approval workflows, making it challenging to enforce human-in-the-loop requirements for critical operations such as database modifications or financial transactions. |
Limited Audit Trails and Monitoring |
Although MCP enables powerful integrations, it doesn't inherently offer comprehensive monitoring of prompts. This can hamper security investigations and compliance reporting by leaving gaps in the recorded interactions. |
Privilege Management Challenges |
Managing access across multiple MCP servers with differing security needs becomes complex. Without proper controls, ensuring that every component operates with the least privilege can be difficult, increasing the risk of overexposure to sensitive operations. |
Secure and Authenticated Model Interaction
One of MCP’s primary benefits is enabling interactions between AI models, MCP servers, tools, and data sources. Traditional “plug-in” style integrations or custom scripts might expose data without robust authentication.
The following table includes some of the key security considerations when implementing the MCP transport mechanisms, along with best practices and implementation notes:
Category |
Best Practices / Considerations |
Description / Implementation Notes |
Authentication & Authorization |
Adopt Standardized Protocols |
- Standard protocols provide a secure framework for managing tokens. |
Data Security |
Use TLS for Network Transport |
- TLS encryption protects data in transit from eavesdropping or man‑in‑the‑middle attacks. |
Network Security |
Implement Rate Limiting |
- Rate limiting and timeouts help mitigate resource exhaustion and DoS attacks. |
Security Considerations when Exposing Tools
When exposing tools, it's very important to implement robust security measures to safeguard against malicious inputs, unauthorized access, and other potential AI application MCP-related vulnerabilities.
The following table shows the best practices, considerations, and implementation notes.
Category |
Best Practices / Considerations |
Description / Implementation Notes |
Input Validation |
- Validate all parameters against the schema |
- Use JSON Schema validators to enforce input structures. |
Access Control |
- Implement authentication where needed |
- Use robust methods (OAuth, JWT) to authenticate users. |
Error Handling |
- Don’t expose internal errors to clients |
- Return generic error messages to prevent leakage of internal system details. |
Additional Security Considerations and Best Practices
The following table lists additional security concerns and key requirements for implementing MCP.
Area |
Key Requirements |
Implementation Considerations |
User Consent & Control |
- Users must explicitly consent to and understand all data access and operations. |
- Provide intuitive interfaces that transparently show which data is being accessed and why. |
Data Privacy |
- Hosts must obtain explicit user consent before exposing user data to servers. |
- Enforce encryption and strong access control policies to safeguard sensitive data. |
Tool Safety |
- Tools represent arbitrary code execution and require caution. |
- Clearly document each tool’s functionality and potential impact to help users make informed decisions. |
LLM Sampling Controls |
- Users must explicitly approve any LLM sampling requests. |
- Ensure the sampling process is transparent by exposing critical details to users before execution. |
Monitoring Agentic Implementations
MCP supports a uniform method for servers to transmit structured log messages to clients. Clients can adjust the verbosity of these logs by setting a minimum severity level, while servers send notifications that include the log severity, an optional logger identifier, and additional JSON-serializable information.
However, when AI agents and applications have the ability to retrieve or manipulate data, maintaining a traceable record of those actions is essential. Cisco AI Defense enhances the security of modern AI implementations (like agents powered by MCP) by providing robust, real‑time monitoring and threat detection that go beyond the protocol’s structured logging. You can learn more about Cisco AI Defense here.
You must be a registered user to add a comment. If you've already registered, sign in. Otherwise, register and sign in.